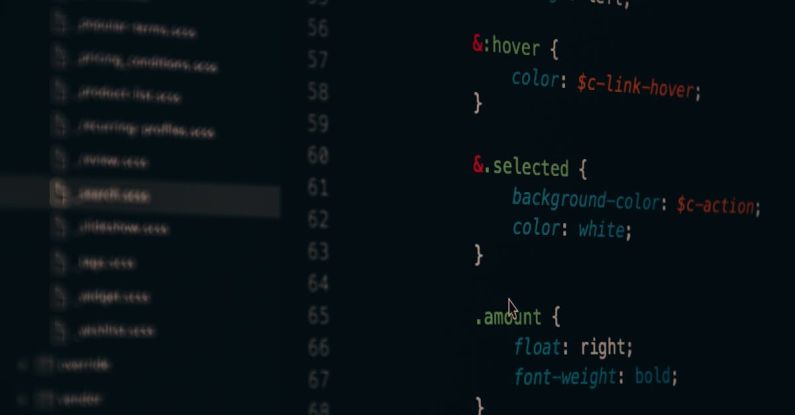
In recent years, the integration of machine learning into robotic systems has revolutionized the field of robotics, paving the way for more intelligent and adaptive machines. By leveraging the power of artificial intelligence, robots are now capable of learning from experience, making decisions, and performing tasks with a level of sophistication that was once thought to be the stuff of science fiction. This article explores the various ways in which machine learning is being integrated into robotic systems, from autonomous vehicles to industrial robots, and the impact it is having on the future of automation.
**Enhanced Sensing and Perception**
One of the key areas where machine learning is making a significant impact on robotic systems is in the realm of enhanced sensing and perception. Traditional robots relied on pre-programmed algorithms to interpret sensory data and make decisions based on predefined rules. However, with the advent of machine learning techniques such as deep learning and neural networks, robots can now be trained to recognize patterns, objects, and environments with a high degree of accuracy.
By feeding large amounts of data into their systems, robots can learn to classify objects, detect obstacles, and even predict future events based on past experiences. This level of enhanced sensing and perception not only improves the efficiency and effectiveness of robotic systems but also enables them to adapt to changing environments in real-time.
**Autonomous Navigation and Control**
Another area where machine learning is being integrated into robotic systems is in the realm of autonomous navigation and control. Autonomous vehicles, drones, and mobile robots are increasingly relying on machine learning algorithms to navigate complex environments, avoid obstacles, and optimize their paths to reach their destinations efficiently.
Through a combination of sensor data, mapping technologies, and machine learning algorithms, robots can now learn to navigate unfamiliar terrain, make split-second decisions to avoid collisions, and even learn from their mistakes to improve their future performance. This level of autonomy not only increases the efficiency and safety of robotic systems but also opens up new possibilities for applications in areas such as transportation, logistics, and search and rescue operations.
**Adaptive Learning and Decision-Making**
One of the most exciting developments in the integration of machine learning into robotic systems is the ability for robots to engage in adaptive learning and decision-making. Traditional robots were limited by their pre-programmed algorithms and rules, making them inflexible and unable to adapt to changing circumstances.
By incorporating machine learning techniques such as reinforcement learning and evolutionary algorithms, robots can now learn from experience, optimize their performance over time, and make decisions based on real-time feedback. This level of adaptive learning and decision-making not only improves the versatility and capabilities of robotic systems but also opens up new possibilities for applications in dynamic and unpredictable environments.
**Future Implications and Challenges**
As machine learning continues to be integrated into robotic systems, the future implications are vast and far-reaching. From the development of more intelligent and autonomous robots to the creation of entirely new applications and industries, the possibilities are virtually limitless.
However, this integration also comes with its own set of challenges. Ensuring the safety and reliability of machine learning-enabled robotic systems, addressing ethical concerns surrounding autonomous decision-making, and overcoming technical limitations are just a few of the challenges that researchers and engineers are currently facing.
**In Summary**
The integration of machine learning into robotic systems is transforming the way we interact with and perceive robots. By enhancing sensing and perception, enabling autonomous navigation and control, and facilitating adaptive learning and decision-making, robots are becoming more intelligent, versatile, and capable than ever before.
As we continue to push the boundaries of what is possible with machine learning and robotics, the future holds exciting opportunities for innovation and advancement. By addressing the challenges and limitations that come with this integration, we can unlock the full potential of intelligent robotic systems and revolutionize the way we work, live, and interact with technology.