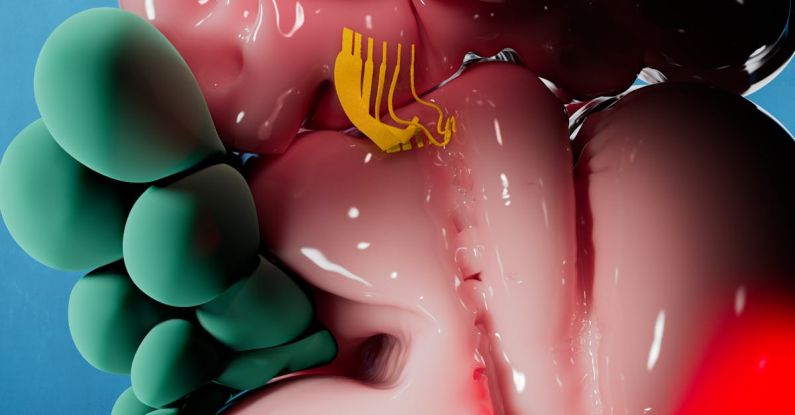
Artificial intelligence and robotic algorithms have become increasingly prevalent in our daily lives, from virtual assistants to self-driving cars. While these technologies offer numerous benefits, there is a growing concern about the potential biases that can be embedded in their algorithms. Addressing bias in robotic algorithms is crucial to ensure fairness and equity in their applications. In this article, we will explore strategies to identify and mitigate bias in robotic algorithms.
Understanding Bias in Robotic Algorithms
Bias in robotic algorithms refers to the systematic errors or unfairness that can occur due to the data used to train these algorithms. These biases can lead to discriminatory outcomes, reinforcing existing societal inequalities. It is essential to recognize that algorithms are not inherently biased, but rather reflect the biases present in the data they are trained on. For instance, if a facial recognition algorithm is primarily trained on data sets consisting of predominantly white faces, it may perform poorly when identifying faces of other races.
Data Collection and Preprocessing
One of the key steps in addressing bias in robotic algorithms is ensuring that the data used to train these algorithms is diverse and representative of the population it will interact with. This involves collecting data from a variety of sources and ensuring that it is balanced across different demographic groups. Additionally, preprocessing techniques such as data augmentation and data anonymization can help reduce bias in the training data.
Algorithm Transparency and Interpretability
Transparency and interpretability of robotic algorithms are essential for identifying and addressing bias. By making algorithms more transparent, researchers and developers can better understand how decisions are being made and identify any potential biases. Interpretability techniques such as model visualization and feature importance analysis can provide insights into the inner workings of algorithms and help identify problematic areas.
Fairness Metrics and Evaluation
Developing fairness metrics and evaluation criteria is crucial for assessing the performance of robotic algorithms in terms of bias. These metrics can help quantify the impact of bias on different demographic groups and ensure that algorithms are making fair and equitable decisions. By continuously monitoring and evaluating algorithms using these metrics, developers can identify and address bias in real-time.
Mitigating Bias in Decision-making
One way to mitigate bias in robotic algorithms is through the use of bias mitigation techniques such as fairness-aware learning and algorithmic adjustments. Fairness-aware learning involves modifying the training process to explicitly account for fairness constraints, ensuring that the algorithm does not discriminate against certain groups. Algorithmic adjustments, such as reweighting or resampling data, can also help mitigate bias by addressing imbalances in the training data.
Diversity in Development Teams
Increasing diversity in development teams is another effective strategy for addressing bias in robotic algorithms. By bringing together individuals from different backgrounds and perspectives, development teams can better identify and understand potential biases in algorithms. Diverse teams are more likely to consider a wide range of societal implications and ensure that algorithms are fair and inclusive.
Ethical Considerations and Accountability
Ethical considerations and accountability play a crucial role in addressing bias in robotic algorithms. Developers and organizations must adhere to ethical guidelines and principles when designing and deploying algorithms to ensure that they do not perpetuate discriminatory practices. Establishing clear accountability mechanisms and oversight processes can help prevent the unintended consequences of biased algorithms.
In conclusion, addressing bias in robotic algorithms is a complex and multifaceted challenge that requires a combination of technical, ethical, and social solutions. By adopting a proactive approach that includes diverse data collection, algorithm transparency, fairness metrics, bias mitigation techniques, diversity in development teams, and ethical considerations, we can work towards creating more fair and equitable robotic algorithms that benefit society as a whole.